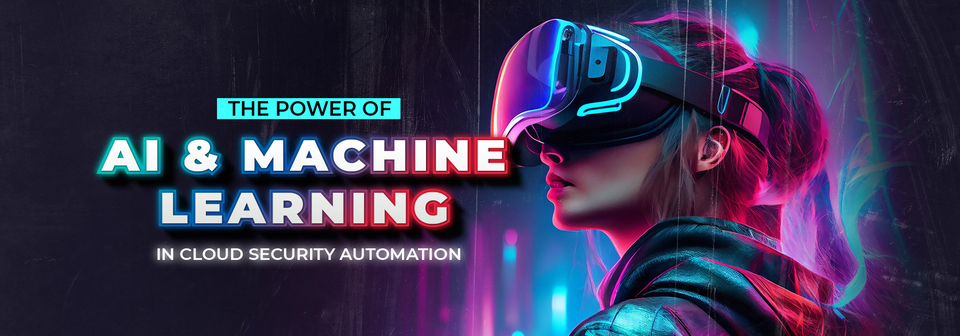
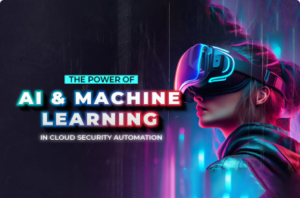
The Power of AI and Machine Learning in Cloud Security Automation
Cloud Security has always been a top priority for organizations that rely on cloud computing. However, traditional security approaches can be time-consuming, expensive, and prone to human error. This is where machine learning (ML) and artificial intelligence (AI) come into play. AI and ML are transforming cloud security by enabling proactive threat detection and response.
In this blog, we’ll explore the power of AI and ML in cloud security automation.
PeoplActive is an ISO 27001:2013 certified leading tech hiring platform. By utilizing an exclusive network of 4000+ Silicon Valley caliber tech talent specialized in 100+ in-demand IT skills, it was pretty easy for businesses to hire game-changing engineers and developers in just 48 hours. So, if you want to accelerate your business, schedule a quick call with our experts now.
How AI and ML are Revolutionizing Cloud Security?
AI and ML have revolutionized cloud security by automating threat detection and response. In the past, security teams relied on rule-based approaches to detect and respond to threats. These approaches were reactive and required human intervention. Security teams may proactively identify threats with AI and ML and take immediate action.
Security teams can now examine massive amounts of data to find patterns and abnormalities that can point to a potential danger. This is made possible by AI and ML. Algorithms that use machine learning can learn from past data to recognize new dangers and adjust to evolving hazards. This enables security teams to identify risks more quickly and react more successfully.
Also Read: Cloudy with a Chance of Risks: Top Strategies for Tackling Cloud Security in 2023
Benefits of AI and ML in Cloud Security
The use of AI and ML in cloud security automation has a number of advantages, such as:
Detecting and responding to threats more quickly
Security teams are better able to respond quickly because of AI and ML’s ability to identify risks in real time. This is critical because it reduces the time that an attacker has to operate within the network. By responding quickly, security teams can prevent attackers from exfiltrating sensitive data or causing other damage.
Reduced False Positives
Traditional security approaches often generate a high number of false positives, which can be time-consuming and frustrating for security teams. AI and ML can reduce the number of false positives by analyzing data more accurately and identifying true threats more efficiently. This allows security teams to focus their time and resources on real threats.
Improved Security Posture
AI and ML enable security teams to improve their security posture by detecting and responding to threats more effectively. This reduces the risk of a successful cyber attack, which can result in data breaches, financial losses, and reputational damage. By improving their security posture, organizations can increase customer trust and gain a competitive advantage.
Use Cases for AI and ML in Cloud Security Automation
There are several use cases for AI and ML in cloud security automation, including:
Anomaly Detection
AI and ML can be used to detect anomalies in network traffic, user behavior, and application performance. By analyzing historical data, machine learning algorithms can identify patterns that may indicate a potential threat. As a result, security teams can respond to attacks proactively and stop them before they cause harm.
Threat Hunting
Threats that might have evaded detection by conventional security measures can be sought out using AI and ML. By analyzing data across the network, machine learning algorithms can identify suspicious activity that may indicate a potential threat. This allows security teams to investigate and respond to threats more effectively.
Incident Response
AI and ML can be used to automate incident response processes. By integrating AI and ML into incident response workflows, security teams can respond faster and more effectively to threats. This can reduce the impact of a cyber attack and minimize the time required to restore normal operations.
Also Read: What is Cloud Security? Why is Cloud Security Mission-Critical?
Challenges of AI and ML in Cloud Security
While AI and ML have significant benefits for cloud security automation, some challenges need to be addressed. These challenges include:
Lack of Expertise
AI and ML require specialized expertise that may not be available within the organization. This can make it difficult to implement and maintain AI and ML-based security solutions.
Data Quality
AI and ML rely on high-quality data to provide accurate results. If the data used to train machine learning algorithms is incomplete or inaccurate, the results may not be reliable.
Security Risks
AI and ML-based security solutions may introduce new security risks. For example, machine learning algorithms may be vulnerable to adversarial attacks that can trick the system into making incorrect decisions. It is important to implement appropriate security measures to mitigate these risks.
Adaptation to Current Systems
AI and ML-based security solutions need to be integrated with existing systems and workflows. This can be challenging, especially if legacy systems are involved. It is important to ensure that AI and ML-based security solutions integrate seamlessly with existing systems to avoid disruptions to normal operations.
Best Practices for AI and ML in Cloud Security
To maximize the benefits of AI and ML in cloud security automation and address the challenges, organizations should follow best practices, including:
Start Small
Organizations should start small and focus on specific use cases that can provide tangible benefits. This allows security teams to gain experience and expertise before scaling up.
Invest in Expertise
Organizations should invest in expertise to implement and maintain AI and ML-based security solutions. This can involve hiring specialized personnel or partnering with external experts.
Ensure Data Quality
Organizations should ensure that the data used to train machine learning algorithms is accurate, complete, and relevant. This requires appropriate data governance and quality assurance processes.
Implement Appropriate Security Measures
Organizations should implement appropriate security measures to mitigate the risks associated with AI and ML-based security solutions. This includes measures such as encryption, access controls, and regular security assessments.
Monitor and Evaluate Performance
Organizations should monitor and evaluate the performance of AI and ML-based security solutions to ensure they are providing the expected benefits. This involves collecting metrics and analyzing data to identify areas for improvement.
Final Thoughts
AI and ML are transforming cloud security by enabling proactive threat detection and response. By automating threat detection and response, AI and ML based security solutions can reduce the time to detect and respond to threats, reduce false positives, and improve the overall security posture of organizations. However, implementing AI and ML based security solutions requires specialized expertise, appropriate data governance and quality assurance processes, and appropriate security measures. Organizations that follow best practices can maximize the benefits of AI and ML in cloud security automation and mitigate the associated risks.
At PeoplActive, we understand that the cloud can be a daunting place, filled with unknown risks and uncertainties. That’s why we offer comprehensive Cloud Consulting Services and On-demand talent-hiring services to help you navigate these challenges with confidence. Our team of expert consultants and talented professionals will work with you to develop a customized cloud strategy that meets your unique needs and goals. With our help, you can rest easy knowing that your company’s data is safe and secure in the cloud.
So why wait?
Let PeoplActive help you take that first step towards a brighter, more secure cloud future today.